APIXIO'S HEALTHCARE TECHNOLOGY PLATFORM
Platform Overview
More than 1.2B clinical and administrative health documents are generated every year, but this data remains siloed and difficult for plans and providers to access and use. Apixio’s proprietary healthcare technology platform unifies data acquisition/management and analysis, patient health profiles, program-specific workflows and services, and optimizes performance with reporting and analytics to help healthcare organizations transform data at scale into actionable insights that improve performance across the healthcare ecosystem.
How It Works
Acquire Data
Transform & Centralize Data
Assemble Profile
Deliver Insights
Optimize Performance
Our Platform’s Impact
$1
billion +
$1 billion+ in identified annual savings
50
million +
50M+ patients analyzed
5x
Faster
5X faster risk adjustment chart reviews
98%+
Accuracy
98%+ accuracy for IBR and DRG validation
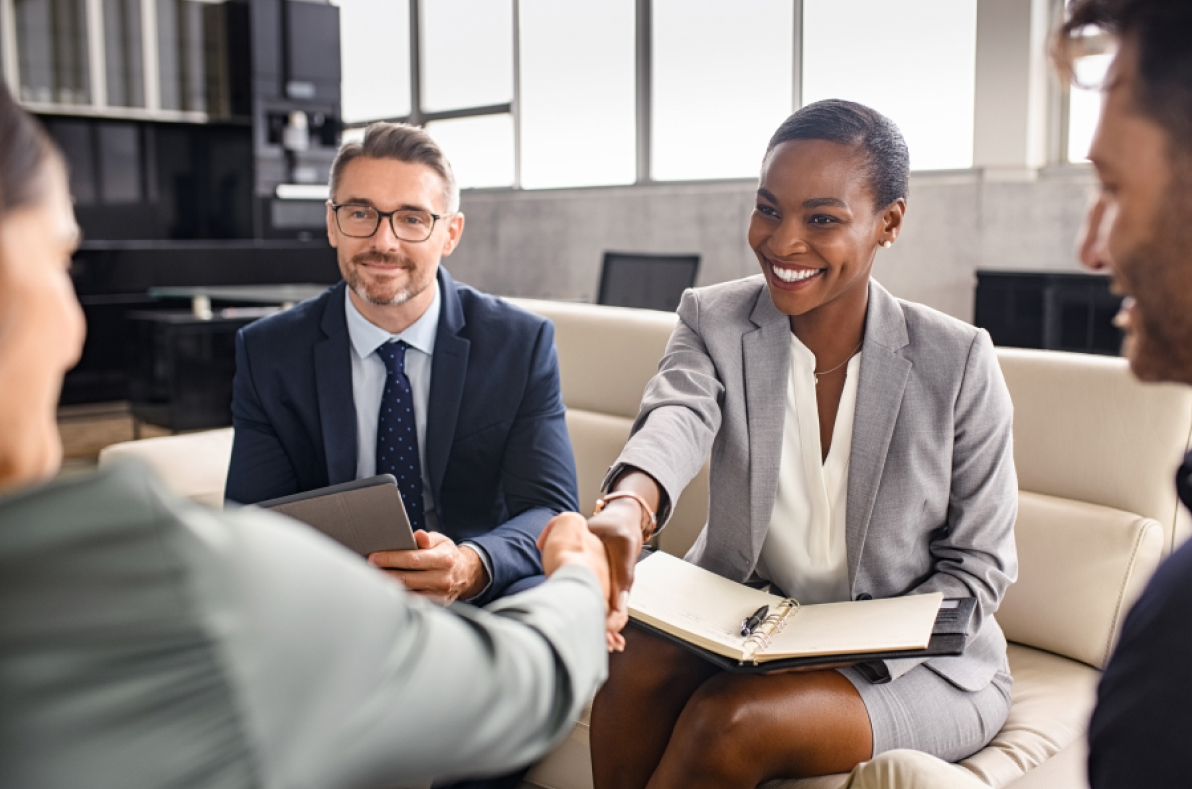
Powerful Technology, Reliable Results
Apixio’s machine learning is great. I’ve seen from personally reviewing records that the platform is spot on with what page and paragraph we need to look at.
Senior Medical Director
Regional Health Plan
Regional Health Plan
Featured Resources
Connect the Dots with Our Connected Technology
Explore how Apixio can support your organization’s unique administrative and clinical program needs.